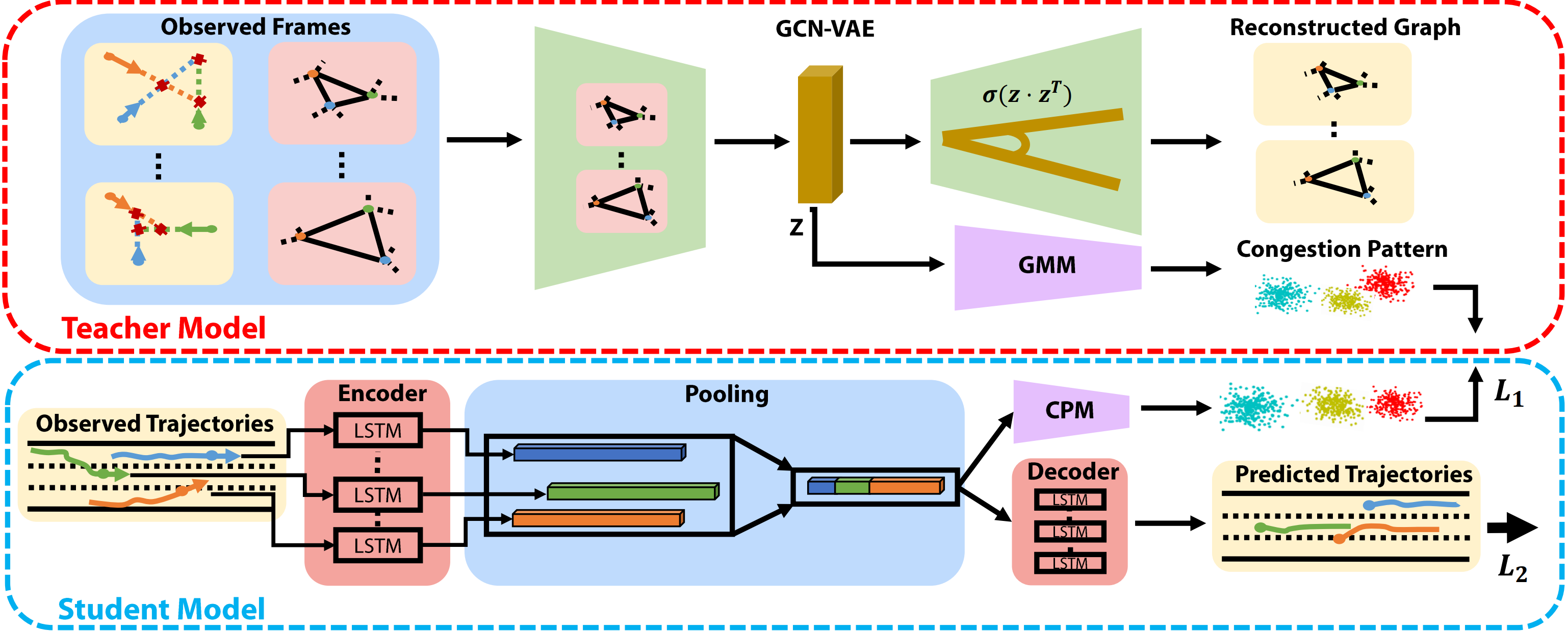
Predicting agents’ future trajectories plays a crucial role in modern AI systems, yet it is challenging due to intricate interactions exhibited in multi-agent systems, especially when it comes to collision avoidance. To address this challenge, we propose to learn congestion patterns as contextual cues explicitly and devise a novel “Sense–Learn–Reason–Predict” framework by exploiting advantages of three different doctrines of thought, which yields the following desirable benefits: (i) Representing congestion as contextual cues via latent factors subsumes the concept of social force commonly used in physics-based approaches and implicitly encodes the distance as a cost, similar to the way a planning-based method models the environment. (ii) By decomposing the learning phases into two stages, a “student” can learn contextual cues from a “teacher” while generating collision-free trajectories. To make the framework computationally tractable, we formulate it as an optimization problem and derive an upper bound by leveraging the variational parametrization. In experiments, we demonstrate that the proposed model is able to generate collision-free trajectory predictions in a synthetic dataset designed for collision avoidance evaluation and remains competitive on the commonly used NGSIM US-101 highway dataset.