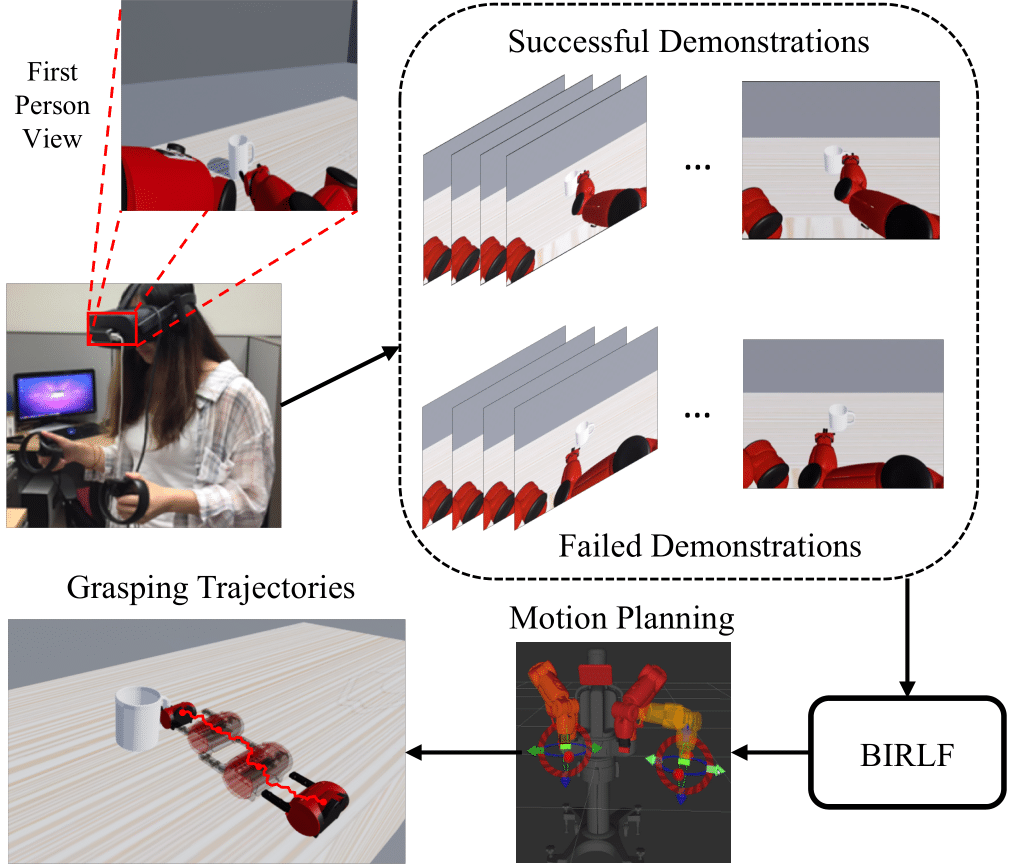
We propose Bayesian Inverse Reinforcement Learning with Failure (BIRLF), which makes use of failed demonstrations that were often ignored or filtered in previous methods due to the difficulties to incorporate them in addition to the successful ones. Specifically, we leverage halfspaces derived from policy optimality conditions to incorporate failed demonstrations under Bayesian Inverse Reinforcement Learning (BIRL) framework. Under the continuous control setting, the reward function and policy are learned in an alternative manner, both of which are estimated by function approximators to guarantee the learning ability. Our approach is formulated as a model-free Inverse Reinforcement Learning (IRL) method that naturally accommodates more complex environments with continuous state and action spaces. In experiments, we demonstrate the proposed method in a virtual grasping task, achieving a significant performance boost compared to existing methods.